Before firms can start their digital journeys, they need to unlock valuable data trapped in documents, PDFs, texts, email messages, and more.
Trapped data has always been the bane of the back office, and the reason so much manual processing remains in middle- and back-office roles.
“For DeepSee.ai, unlocking that data is the key to success as firms look to accelerate their move toward full-scale digital transformation,” says Steve Shillingford, CEO of DeepSee. “Much of the most valuable data in the middle and back office of the bank is in an unstructured format: Word documents, PDFs, texts, and emails, emails, emails everywhere.”
By focusing on unstructured data, Shillingford argues, DeepSee’s artificial intelligence-driven platform can automate the most time-intensive data processes in a financial services firm without disrupting other well-established data flows.
“If it’s in an email, if it’s in a messaging system like Symphony, or if it’s in a fairly older, later generation system, we can plug into that very simply without touching the infrastructure, and mine that data without creating destruction,” he says. “We can do that in a compartmentalized way — it accelerates the ability to get at the actual value that’s under the covers.”
With a background in building large-scale data surveillance platforms for government agencies and Fortune 500 organizations, Shillingford has deep experience mining and performing various levels of analytics and forensics on unstructured content. In financial services, he saw an opportunity to implement that same compartmentalized approach to unstructured data analysis because of the high number of manual middle- and back-office processes financial firms have, and the associated complexity of the highly regulated environment in which the financial industry operates.
The approach has begun to gain attention.
In a May report titled Cool Vendors in Conversational and Natural Language Technologies, market research firm Gartner characterized the company’s platform as technology that “might look like a text extraction platform but delivers a new breed of unstructured data management and processing solutions.”
A cloud-based, A.I.-driven platform, the system is easy to roll out, according to Shillingford.
In fact, DeepSee has already “taught” financial, insurance, and legal terms to its BERT transformers, a version that the company has dubbed “FILBERT.” (Bidirectional Encoder Representations from Transformers, or BERT, is a technique for natural language processing pre-training that was developed by Google.)
“As a result, the DeepSee software is optimized to provide users big data answers, using small data sets, eliminating the challenges of training typical machine learning models,” Shillingford says.
This training can be easily done by a business user or subject-matter expert at a bank without programming skills, according to Shillingford.
A.I. Driven by Business Users
Setting up the DeepSee system involves first identifying the data that will be mined and the business process that will be automated, Shillingford says.
DeepSee and the client then agree to the point in the process where a subject-matter expert will observe and fine-tune the data mining process. The subject-matter expert uses a point-and-click, no-code tool to train and tune the algorithm, by accepting, rejecting, or modifying the data dispositions that the system identifies. It is no more complicated than cutting and pasting on an Apple iPhone, he says. Reconciled data is then sent downstream to whichever system needs it.
Shillingford estimates that it takes 30 to 90 days to set up and establish a workflow process using the platform. Guided by a business user, the system learns quickly to identify the types of terms that the user is looking for, cutting processing times and error rates.
“Repeat that process several hundred times, or several thousand times, and all of a sudden the machine goes from let’s say 95 percent accurate to 99 percent and, in some cases, 100 percent accurate,” he says.
Artificial intelligence and machine learning techniques generally require massive data sets to “learn” to identify the required information. Having a human business-user guiding the process enables the algorithm to learn with a significantly smaller data set, Shillingford says.
“What we’ve optimized is the ability to do big data analysis with small data sets,” he says.
Opportunities & Resistance
In the back office, typical use cases for the technology include tasks like reconciliation, compliance, and corporate actions processing, Shillingford says.
“Any business flows where you have reconciliation of one-to-many types of data sets, those are great examples of how to automate and really get a lot of value, because you can take those down from hours to minutes, and we can take the error rates from 10, 20, 30 percent down to zero pretty easily,” he says.
Some firms use the technology to reprice risk models, and recently, DeepSee is seeing increased interest in applying its technology toward the ongoing transition away from the London Inter-Bank Offered Rate (LIBOR).
“Those are great use cases in the sense that it gives you a nice historical lens into what happened, and it also gives you a little bit of practice into how things will look going forward without actually putting it into production,” he says.
A challenge for companies like DeepSee is that the middle- and back-office space has historically been slow to adopt new technology, analysts say.
“In the front office, people are more likely to experiment and test use cases, but the back office has more of an attitude that we don’t want to fail and can’t make things worse, so let’s go slowly,” says David Easthope, a senior advisor at Coalition Greenwich, a market research firm.
That risk-averse attitude can make the back office a tough place for vendors to break into.
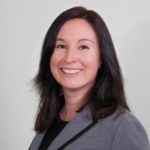
Virginie O’Shea
“Any new entrant to this space finds it challenging to gain traction because of the relatively conservative nature of large firms’ vendor risk assessment teams,” says Virginie O’Shea, founder of Firebrand Research.
Privately, some firms have expressed reluctance to adopt advanced technologies in the back office because their data isn’t “ready” yet, according to Coalition Greenwich’s Easthope.
“They aren’t collecting all the data they need. Sometimes it’s in different data siloes. It has to be formatted. There is still some enrichment that needs to be done. You discover a lot of manual things that you do,” Easthope says. “They say look, we need to clean up our data first.”
But Shillingford sees those types of data issues and manual processes as opportunities for DeepSee.
“If Becky over in finance is the only one who knows how to handle this reconciliation, and she’s gone that day, or she quit, that knowledge is never captured,” he says. “That’s why we call it ‘knowledge process automation,’ because you can build that knowledge into the model and actually create organizational IP [intellectual property] by virtue of automating this,” Shillingford says.
One of the firms using DeepSee for post-trade processing and reconciliation cut review time per transaction from between 60 and 90 minutes, down to two-to-three minutes. Average daily transaction throughput increased tenfold, from six transactions per day to 60 transactions per day. The average review time shrank by 80 percent, from T+30 down to T+5.
Dramatic results like that can be achieved, according to Shillingford, precisely because of the manual and disjointed nature of some of the processes that the system is targeting. In some cases, he says, existing processes involve manually finger tracing between two or three different screens to compare data.
“Imagine automating that. Machines don’t make those kinds of mistakes, so that is one way you get immediate ROI,” he says.
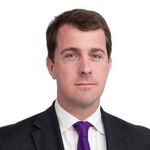
David Easthope
One recent incentive for automating back-office processes has been regulatory pressure, Shillingford says. When the volatile “meme” stock trading exploded at the start of 2021, several clients expressed concern that regulators were pushing for faster settlement, he says.
“Going from T+2 to T+1 or from T+1 to T+0 is not 100 percent more difficult, it’s orders of magnitude in difficulty because of all the other processes that they have not yet automated, so there is a lot of interest and urgency in getting ahead of that,” he says.
The Pandemic Effect
Meanwhile, starting in March 2020, a new threat began driving increased interest in back-office automation, according to Shillingford.
Manual back-office processing functions that may have been outsourced to areas with lower-cost labor forces, became increasingly difficult to manage when the COVID-19 pandemic forced workers around the world to leave the office and work remotely.
“All those strategies where you could outsource a lot of this to low-cost labor geographies went out the window,” Shillingford says. “Automating this just makes having people working from home more effective, and frankly more productive. So, we hear a lot of people starting to say I have to take advantage of some of these low-hanging fruit opportunities, because who knows?”
In addition to mitigating the risk of workflow problems caused by external circumstances, Shillingford maintains that automating manual processes with machine learning technology enables firms to capture and preserve institutional workflows.
“You want to automate not only because it gives you an ROI, and makes running your operations less expensive, and you’re faster to capture customers, but you have a lot of institutional knowledge that is difficult to surface,” Shillingford says.
Accessing that institutional knowledge, or “knowledge mining” can bring increased visibility into processes that competitors might not have the same ability to see, he says.
“All of a sudden you have insight that customers and other peer firms don’t have access to,” Shillingford says. “You are more competitive in terms of understanding what new products to offer, or what opportunities might exist, and obviously, that’s the holy grail.”
Need a Reprint?