Hudson River Trading’s collaboration with Google Cloud could be good news for post-trade operations.
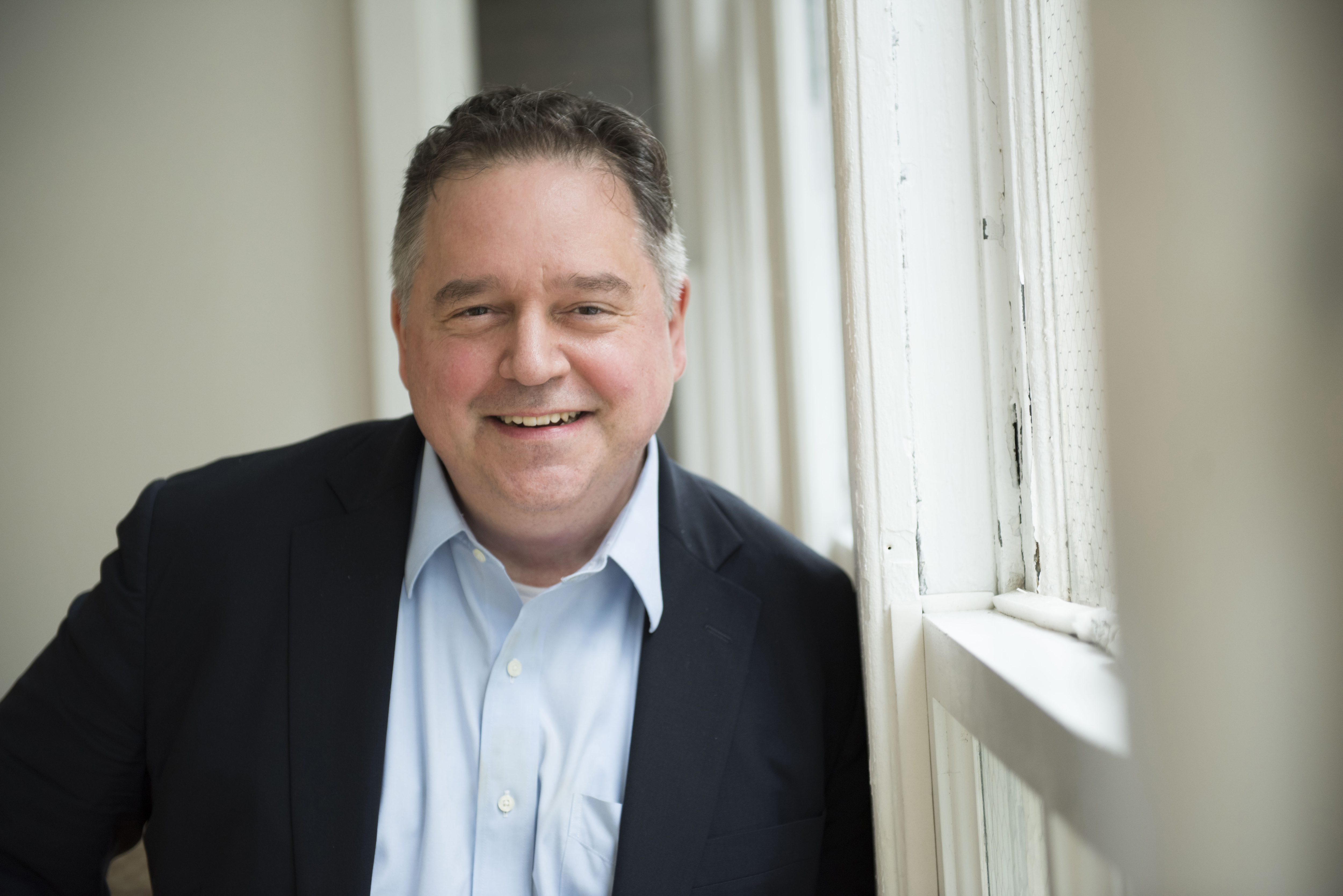
Grygo is the chief content officer for FTF & FTF News.
We at FTF and FTF News focus much of our attention on post-trade operations.
But every once in a while we take a look at some of the breakthroughs happening in the front office. Such advances ripple through to the middle- and back-office Ops teams.
A case in point is the quantitative trading firm Hudson River Trading (HRT) and its recently announced collaboration with Google Cloud.
The collaboration was formed to speed up the development of HRT’s automated trading models, officials note. In fact, the HRT effort builds upon Google Cloud’s previous collaborations with market makers Citadel Securities and Two Sigma.
Based in New York City, HRT is a multi-asset class quantitative trading firm that develops automated trading algorithms to facilitate the search for liquidity and price discovery via securities exchanges and alternative trading systems, officials say. The firm, founded in 2002, has offices around the world and trades equities, futures, options, currencies, and fixed income in 200 markets globally.
Officials say that the firm needs to analyze “vast amounts” of financial data and execute trades for its algorithms and models. Thus HRT will be using Google Cloud’s infrastructure to “test new strategies in a shorter amount of time in a cost-effective manner, allowing them to better react to the market,” according to the announcement.
As noted earlier, researchers at HRT will be able to access Google Cloud’s artificial intelligence (A.I.) computer chips and intelligent workload scheduling tools. Researchers will be able to test A.I. chips such as NVIDIA GPUs, and to run complex research workloads and trading simulations at scale.
“HRT is empowered to efficiently iterate on complex trading models and simulations,” officials add.
The Google Cloud collaboration provides HRT with a platform that allows the firm to “rapidly iterate on ideas, with tooling and vast resources to enable a fast path from inception to scale-out production,” says Gerard Bernabeu Altayo, compute lead, at Hudson River Trading, in a prepared statement.
One of the key aspects of this collaboration that may translate into post-trade operations is the Google Cloud Dynamic Workload Scheduler offering and Spot Virtual Machines that are “playing a crucial role in optimizing HRT’s compute resources,” officials say.
Officials say that the workload scheduler and spot virtual machine capabilities can enhance access to graphics processing units or GPUs. High-performance GPUs on Google Cloud are “for machine learning, scientific computing, and generative A.I.,” according to Google Cloud.
A range of GPU types are used via Google Cloud: “NVIDIA H100, L4, P100, P4, T4, V100, and A100 GPUs provide a range of compute options to cover your workloads for a broad set of cost and performance needs,” according to Google Cloud officials. “Optimally balance the processor, memory, high-performance disk, and up to 8 GPUs per instance for your individual workload. All with the per-second billing, so you only pay only for what you need while you are using it.”
The Dynamic Workload Scheduler allocates A.I. chips based on real-time demand, with the intention of efficiently using compute capacity.
It’s not a stretch to think that some of the Google Cloud tools that HRT is using to optimize compute resources for quant trading could be used for post-trade securities operations.
In February 2021, BNY Mellon and Google Cloud announced that they were collaborating to improve U.S. Treasury Market settlement and clearance processing. The solution built via Google Cloud was intended to help BNY Mellon clients forecast “40 percent of daily settlement failures to improve overall market liquidity,” officials said at the time.
The solution also targeted BNY Mellon market participants who were able to “generate significant capital and liquidity savings and unlock operational efficiencies.” The initiative leveraged Google Cloud’s data analytics, A.I., and machine learning (ML) technologies for developing collateral management and liquidity solutions.
Over the past three years, it’s clear that post-trade systems are moving fast to adopt more A.I.-based technologies. It’s also clear that A.I. will take a lot of computing resources, which means that firms will need new ways to keep costs down
Need a Reprint?