Todd Sloan at Electra tells FTF News that the next release of the vendor’s flagship offering will feature machine learning and a fuzzy matching algorithm.
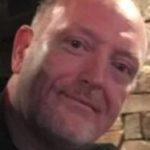
Todd Sloan
(Electra Information Systems, Inc., a provider of reconciliation and other post-trade solutions, won the 2019 FTF News Technology Innovation award for Best Reconciliation Solution, its fourth consecutive win in this category. FTF News got time with Todd Sloan, senior vice president, business development and product management for Electra. Sloan joined Electra in 2003 as a pre-sales engineer overseeing proofs of concept for Electra’s flagship product, Electra Reconciliation. He was promoted to vice president of Electra’s consulting team in 2005 after managing reconciliation projects for AQR Capital, GlobeFlex, Axiom International, Dodge and Cox, DuPont Capital, Bridger Capital, and others. Before Electra, Sloan served as a project manager at Citibank and worked for SmartStream Technologies.)
Q: On average, how much time do firms spend on investigating trade breaks?
A: The amount of time it takes to investigate trade breaks depends on the complexity and volumes of the instruments and markets being traded. But, overall, the process is time-consuming because firms must rely on the brokers to email or call when they see an unmatched or failing trade, as well as rely on reporting from custodians that may or may not be in real time.
It takes time to gather all the information across custodians and brokers, then organize it into a format that is viewable. Right now, most of the communication and transfer of information is done through email, which causes issues to be easily missed.
After identifying exceptions, the real work begins as portfolio accountants scramble to uncover the root-cause and a solution for each break.
The investigation process often involves interacting with many different data sources outside of the reconciliation solution. These data interactions may include accessing individual custodian websites and other data terminals such as Bloomberg. Each of these external interactions in the investigation process increases the amount of time spent resolving breaks.
Q: What was driving the changes that were made to Electra Reconciliation during 2018?
A: After taking a closer look at the investigations process of our clients, Electra determined that significant improvements could be made if we were able to incorporate the research material being used by portfolio accountants.
This revelation led us to expand our Electra Data catalog to include most of the reports being accessed during the investigations process. Information such as corporate actions, failed trades, collateral and security lending plays a vital role in researching exceptions.
By leveraging our patented, intelligent integration capabilities, Electra Reconciliation can dramatically expedite the investigation of breaks by dynamically assisting the accountant with the root-cause analysis. This essentially means that whenever the end-user selects an exception, Electra Reconciliation will access its research repository for the relevant information related to the selected break.
By doing so, the end user spends far less time outside of the application accessing external data sources such as a bank website or Bloomberg terminal.
Q: What kinds of new IT were used to expand the features and functions of Electra Reconciliation?
A: The next release of Electra Reconciliation adds machine learning (ML) for external mapping and research, as well as a proprietary fuzzy matching algorithm, designed to address common security identification issues that emerge when reconciling esoteric asset classes lacking exchange-traded identifiers.
If you look at the reconciliation process, an investment manager takes in external files, recognizes them, imports them, compares the information they’ve imported using a set of rules, and produces an output. We’re now adding ML at different stages of the external mapping process to recognize patterns of repeated behavior in order to efficiently present information and prevent duplicate effort.
We’re also adding ML into Electra Reconciliation’s research capability. When an external event occurs in which data is available from a third-party source, the system will recognize when certain kinds of behaviors happen and enable the investment manager to take certain actions with that data which is continually enhanced with ML.
It’s important for investment managers to improve their processing with rule-based systems. But one of the major problems is that data is often dirty, ambiguous or missing.
One example is when an investment manager is missing the appropriate security identifier for a position. With fuzzy matching, Electra Reconciliation will look at other fields beyond identifiers, such as descriptions, to find the best matches on securities.
Leveraging our deep knowledge and experience in the financial services industry, we have designed our fuzzy matching algorithm to be semantic-based, rather than syntactic-based which is common among other vendors. Rather than looking at just characters without underlying meaning, we look at the descriptions to gain an understanding of their meaning, and use the meaning to determine matches.
Q: How do you view the emerging disruptive technologies of artificial intelligence (AI) and ML?
A: The ultimate goal of all the work that Electra does is to reduce the number of exceptions that people need to look at, and reduce the amount of time it takes to look at them.
ML and AI are simply tools that allow us to recognize behaviors and patterns, and integrate those patterns into the workflow seamlessly. As a result, the time it takes for users to do their work continues to decrease, driving greater effectiveness and productivity of employees.
An important thing to mention is accuracy in the realm of ML and AI. We’ve had conversations with firms who are faced with shockingly high human error rates.
When an investment manager is faced with large numbers of exceptions, people simply cannot process them and start to make a lot of errors. Once you have a chance to review something and identify a pattern, and then integrate that into the workflow automatically, then your error rate goes down.
Computers are great at repeating things over and over again, but humans are not. ML and AI are greats tools for identifying patterns and letting a machine do it.
In business, there is a famous triangle of three choices: faster, cheaper and better. Normally, you have to choose two out of the three because they compete with one another. These technologies are disruptive and give you all three: the trifecta.
Q: Among the issues that firms have to worry is net asset valuation (NAV). What is the process for arriving at a definitive NAV?
A: Most investment managers find NAV to be very challenging when using off-the-shelf reconciliation products that often lack the flexibility to accommodate the unique requirements of this specialized reconciliation process.
Electra Reconciliation fully supports NAV reconciliation. But prior to this, most investment managers were stuck with an inefficient, error-prone process that required them to manually gather and populate custodian or fund administration NAV data in their Excel spreadsheets.
Unlike Excel, where data must be manually inserted without a proper audit trail, Electra Reconciliation imports, normalizes, enriches and matches NAV data using a complete audit trail covering all system and user activity.
Electra Reconciliation uses a powerful rules engine for placing all NAV data in categories or buckets, which are then summed up to show the total NAV values on all sides. Users can quickly determine the reason for any discrepancy at the NAV bucket level by drilling into the underlying data that was used to derive the NAV values.
Whether the NAV difference is attributed to a pricing issue or a timing difference, Electra Reconciliation users are fully aware of the issue and how it is being managed from an exception management perspective.
Firms using Electra Reconciliation also benefit from the fact that our Electra Data team has identified the very best NAV data feeds from more than 1,300 custodians.
Q: Have failed trades that cause problems across reconciliation, settlement and trading teams, actually helped firms to break into data silos?
A: Investment management firms typically treat reconciliation and exception management processes as departmental functions, which creates redundancy resulting in increased errors, low staff productivity, and unnecessary operational risks and overhead costs.
With different people researching the same problem, this multiplies the workload and cost for each exception.
In almost all cases, operations staff perform each of these reconciliation processes using different platforms including Excel, system modules or add-ons, and reconciliation-specific applications. Another big problem with this inefficient approach is the absence of enterprise-level investigation audit trails due to their disparate locations. This creates redundancy and unnecessary steps in the investigations process.
In a silo-based reconciliation environment, staff will find themselves duplicating much of the work that had already been performed by their colleagues. To make matters worse, the repetitive investigation work performed across different teams is often hidden from management, so the inefficiency goes undetected.
As transaction volumes increase, inefficiencies will become apparent in the form of scalability issues and the need for more staff.
To address this issue, Electra Reconciliation supports all the reconciliation processes found across the middle- and back-office functions while also fostering collaboration across these areas.
Rather than viewing multiple reconciliation silos, management and operations staff are able to access a single point of reference where the benefits of collaboration are obvious throughout the investigation’s workflow.
Using the example of a failed trade, the settlements team can work on failed trades where all their actions, notes and documentation are completely visible to other individuals who are interested in knowing the impact of a failed trade. Electra Reconciliation quickly shows how the tentacles of a failed trade may reach a NAV, security lending or corporate action (eligible portion), as well as positions, transactions, or cash balance reconciliation processes.
Q: What kinds of standards and best practices would make exception management and trade reconciliation a less burdensome process?
A: This question is about an aspiration.
SWIFT was standardized as a solution to data exchange. But, in the end, SWIFT data is incomplete and Electra maintains a data delivery service because the quality of the standard is insufficient to the task of matching.
Data continues to be “dirty”: it is incomplete or contains errors. Electra maintains its lead in the market because of a recognition of these weaknesses and its ability to solve the real word problems that come in the financial industry because different systems have different conventions.
Machine learning, fuzzy matching and automatic research integration all work towards a single goal: reduce the time it takes to know where you stand and cut a NAV.
Need a Reprint?